FEATURE
Teaching Digital Literacy in the Context of AI Text-to-Art Generators
by Neil Dixon
AI text-to-art generators democratize the creation of images and art. To generate an image, the user simply enters a text string that describes the content of the image, along with the style, resolution, and features. Prominent services (see Figure 1, Figure 2, and Figure 3) used by artists include DALL•E 2 (OpenAI 2022), Midjourney (Mid-journey 2022), and Stable Diffusion (Hugging Face 2022a). These technologies are so powerful, it is easy to make quick assumptions about how they may change our relationship with art. To be better-informed about the role of AI text-to-art generators in society, digital literacy can promote consideration of the technology’s risks, capabilities, and limitations. In this article, I describe my experience of teaching digital literacy to second-year undergraduates at Anglia Ruskin University (ARU). The article gives a brief overview of digital literacy in the context of AI text-to-art generators and outlines how you can teach students about the technology.
Examples of
AI-Generated Images |
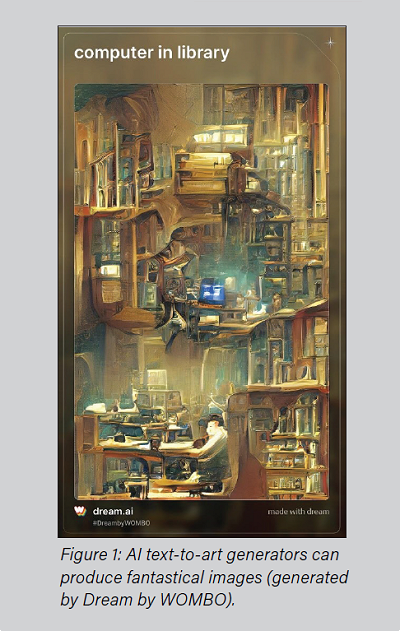 |
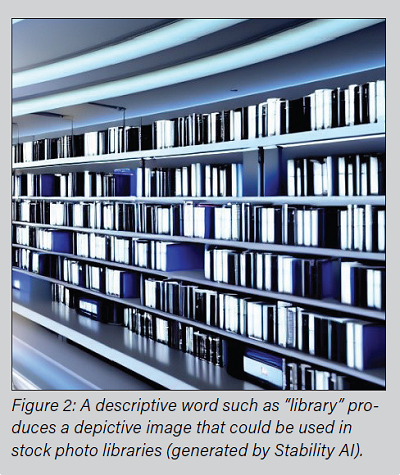 |
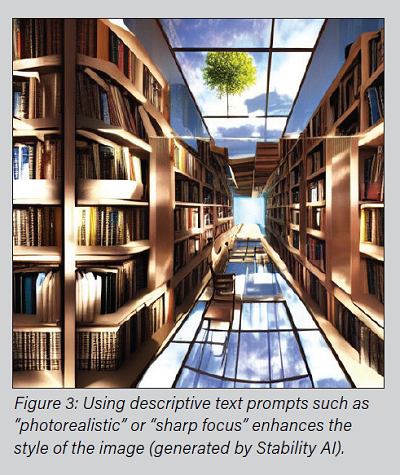 |
Digital Literacy and AI Text-to-Art Generators
Digital literacy means a person has sufficient knowledge and education to operate in the digital world (Goodfellow 2011). Our digital world increasingly includes algorithms, deep learning, machine learning, and neural networks. These technologies and their relationships are so complex that the way they function is outside most people’s understanding—we can only evaluate AI on the outputs it creates. However, awareness of some technical detail can assist us with explaining and evaluating the outputs. A relevant innovation, for example, is a development in machine learning called a diffusion model. This is a generative model that attempts to understand the text input from the user, then map the generated image to reference images (Bouchard 2022). The knowledge builds our understanding that different diffusion models output different art styles, ranging from illustrative to photorealistic.
Providing time to experiment with the technology is another way to develop or enhance digital literacy. We can improve our understanding of the capabilities and limitations, which means we can see the technology from the perspectives of artists, legislators, or developers. This bolsters our ability to debate solutions about issues that arise from using AI text-to-art generators, such as fair use of image likeness, so we can better utilize AI text-to-art generators in a way that is useful in our digital world.
Digital Literacy Lessons
In September 2022, I did two digital literacy lessons on AI text-to-art generators with my second-year undergraduates. Thirty-seven students took part, most of whom were new to AI-generated art. The first lesson was hands-on and designed to develop students’ digital literacy through a practical exercise and discussion. The second lesson was intended for students to get a sense of the capabilities and limitations of AI text-to-art generators and consider the impact of AI-generated images in the digital world.
Lesson 1—Practical Exercise and Discussion (60 Minutes)
- Try an online AI text-to-art generator (15 minutes)—Direct students to a free, online service such as a demo of Stable Diffusion (Hugging Face 2022b), Craiyon by OpenAI (Craiyon 2022), or Dream by WOMBO (WOMBO 2022). Alternatively, you can use the search engine Lexica (Lexica 2022), which contains images produced by Stable Diffusion.
- Think of a specific use of the technologies (2 minutes)—This is a lateral creative thinking exercise to encourage students to think of alternative functions of the technology beyond just “art.” Students could contribute ideas such as marketing copy, comics, magazine covers, billboard advertising, greetings cards, posters, or computer games.
- Discuss issues around AI text-to-art generators (25 minutes)—Arrange students in small groups to discuss the questions that follow. The goal of this activity is to encourage students to share their perspectives and explain how they would solve many of the challenges faced in the digital world by artists and art consumers.
- How are these technologies changing what it means to be an artist?
- What good comes from these technologies?
- What dangers or potential risks do you see about future applications of this technology?
- What are the ethical and legal implications that need to be considered?
- How can we control the production of AI-generated images/art?
Lesson 2—AI-Generated Art in the News (20 minutes)
Students can do this exercise alone or in small groups.
- Read a news article about the impact of AI-generated images or art (10 minutes)—Supply a short news article about AI-generated images or art using a resource such as the AI Incident Database (AI Incident Database 2022). A search for terms such as “deepfakes” or “AI images” produces many relevant incident reports.
- Gain comprehension of the issues discussed in the article (10 minutes)—The questions that follow can be discussed by students in small groups or worked through alone. They are intended for students to extract facts from the article, for example, distinguishing among the reported technologies such as deep learning or algorithms, brand names of the companies involved, and the actors in the story. Another aim is to see that students understand the contexts in which the technology is used and to recognize that the technology’s capabilities and limitations often depend on this context.
- Describe the specific technology: Does it have a name, who provided it, and what kind of other technologies did it use?
- Why do you think this technology was introduced?
- What problems or issues were raised about the technology?
- Which of these issues were unexpected or unintended?
At the end of each lesson, I spent around 10 minutes giving feedback on the answers the students submitted. I did this by highlighting the most insightful comments from the class and suggested ideas where responses required more detail. I pointed out misconceptions, but in most cases, I was careful to emphasize that all responses were valid, and there was not necessarily a right or wrong answer for most questions.
What Students Said During the Lessons
I took notes on student responses to help identify how they conceptualized AI text-to-art generators. The word cloud in Figure 4 on page 22 shows the most prominent keywords from their comments, which included copyright, filtered, legal, violent, jobs, and creativity. Following is a discussion of student views in more detail, which I categorized into three themes I talked about in the introduction: evaluating artistic outputs, the role of AI text-to-art generators in the digital world, and the limitations and capabilities of the technology.
Evaluating artistic outputs — Students raised concerns around the potential dissemination of dehumanizing art, such as violent or sexually explicit images. Comments showed that the risk was more pronounced if the AI-generated art was used in the context of deepfakes or fake news or to promote illegal ideologies. The suggestions to mitigate these risks included implementing filters to censor inappropriate images or introducing checks such as captchas so images were only posted online by a human.
For students to better evaluate the outputs of AI text-to-art generators, it is worth emphasizing the limitations of diffusion models. One limitation is that the technology is only replicating what humans have already produced. This presents a more balanced view that AI text-to-art generators are just another tool for creating artistic outputs. In addition, it reveals positive ways AI text-to-art generators are utilized, including comics and concept art, which also shows that significant work is still required to obtain usable images (Herrman 2022).
Role of AI text-to-art generators in the digital world — Students commented on the ethical and legal issues, such as the ease with which AI text-to-art generators could be used to plagiarize the style of artists or to replicate copyrighted images and intellectual property. Students observed that consent should be obtained from artists before their work is used to train the algorithms of the AI text-to-art generators, and artists should be monetarily compensated. Suggestions to maintain proper ethical and legal use of images centered on improving ways of governing existing copyright legislation. This included watermarking images, so it is clear they were produced by AI, or using technologies such as non-fungible tokens (NTFs) to assist with the identification ownership of copyrighted images.
It is important to facilitate ways for students to be open to new ideas about ethical and legal issues. One way to do this is to pose debates on topics such as, “Should art be a social benefit?” “What if intellectual property functioned like patents?” or “Should we have more liberal copyright legislation?” At the end of the debate, take the most radical ideas and ask the whole group to vote on what they think is the best alternative. This is a good way to assist students in seeing different perspectives and developing their own problem-solving skills.
Limitations and capabilities of the technology — Students responded that AI text-to-art generators could be used to test creative ideas, expand techniques, and help realize artists’ imagination. Due to these enhanced capabilities, students said many artists would make unoriginal art and become more reliant on machines, and the skills in producing hand-drawn artwork could be lost or become less popular. Most students also said AI could take job roles, while only a minority of students said jobs would be created.
The literature shows that people who know less about AI tend to fear the impact (Sowa, Pzerglasky, and Ciechanowski 2021). In this case, it’s the impact on job roles or other art styles. One solution is to help students gain a wider perspective on the history of AI. Cade Metz’s book Genius Makers: The Mavericks Who Brought AI to Google, Facebook, and the World is an engaging read on the progress made on machine learning over the decades, told through the stories of leaders in academic fields and, more recently, Deep Mind, Baidu, and Google. Knowledge such as this informs students about the resources required to develop deep learning and neural networks and helps us realize that AI will not wholly be replacing artists any time soon. Skills such as drawing by hand will still be needed.
Conclusion
These lessons were a useful way to teach digital literacy of AI text-to-art generators for those new to the technology. What worked was the practical experience of the technology, exposure to different ways AI text-to-art generators were used, and opportunities to discuss the issues. The questions and exercises could equally be applied to other AI-generative technologies, such as creative writing or coding. I did learn there was a tendency for students to adopt homogenous views when discussing the questions, so I continue working to enhance confidence and knowledge to take the contrarian approach.
|
Resources
AI Incident Database, 2022. incidentdatabase.ai.
Bouchard, L. 2022. “Google Brain’s Answer to Dalle-e 2: Imagen.” louisbouchard.ai/google-brain-imagen.
Goodfellow, R. 2011. “Literacy, Literacies and the Digital in Higher Education.” Teaching in Higher Education, 16, pp. 131–144.
Herrman, J. 2022. “AI Art Is Here and the World Is Already Different.” Intelligencer, 19 Sept. 2022. nymag.com/intelligencer/2022/09/ai-art-is-here-and-the-world-is-already-different.html.
Hugging Face, 2022a. Stable Diffusion v1-4 Model Card. huggingface.co/CompVis/stable-diffusion-v1-4.
Hugging Face, 2022b. Stable Diffusion 2 Demo. huggingface.co/spaces/stabilityai/stable-diffusion.
Lexica 2022. Lexica: The Stable Diffusion Search Engine. lexica.art.
Metz, C. 2021. Genius Makers: The Mavericks Who Brought AI to Google, Facebook, and the World. Dutton.
OpenAI, 2022. DALL•E 2. openai.com/dall-e-2.
Sowa, K., Przegalinska, A., and Ciechanowski, L., 2021. “Cobots in Knowledge Work: Human–AI Collaboration in Managerial Professions.” Journal of Business Research, 125, pp. 135–142.
Stable Diffusion Online, 2022. Stable Diffusion Playground. stablediffusionweb.com/#demo.
WOMBO, 2022. Dream by WOMBO. wombo.art. |